Artificial intelligence (AI) has undergone tremendous advancements in recent years, and language models are at the forefront of this evolution. From enhancing customer interactions to enabling more intuitive digital tools, AI-powered language models like GPT-4, Gemini, and LLaMA 3.1 have dominated the global conversation. However, a new player has emerged in the Indian AI landscape—SUTRA, a language model specifically designed to excel in Indian languages. Unlike its counterparts, which focus primarily on English and a few other international languages, SUTRA has mastered the nuances of over 20 Indian languages, placing it miles ahead of competitors when it comes to performance in this region.
But what makes SUTRA unique? Why has it managed to outperform renowned models like GPT-4, Gemini, and LLaMA 3.1 in Indian language processing? To understand the impact of this breakthrough, we’ll dive deep into SUTRA’s capabilities, its revolutionary approach to Indian language processing, and why it’s poised to reshape how businesses and organizations operate in multilingual environments like India.
What is SUTRA?
SUTRA is an advanced AI language model developed with a singular goal in mind: to cater to the intricate, diverse, and culturally rich languages of India. The name “SUTRA” is not just a branding strategy; it reflects its function—SUTRA stands for “System for Unified Text Representation and Translation in Asian languages,” with a particular focus on Indian dialects.
A Revolutionary Language Model for Indian Languages
India is a country with over 1.3 billion people, and it boasts more than 1,600 languages and dialects. As a result, linguistic diversity is a key characteristic of the country.
Most global AI models have struggled to support the diverse array of languages, but SUTRA bridges that gap. It draws its training datasets from a rich repository of Indian languages, allowing it to deliver accurate translations, generate language, and understand context in regional languages like Tamil, Telugu, Malayalam, and Bengali.
Traditional AI models often lack sufficient training data in regional languages, limiting their effectiveness. In contrast, SUTRA trains its deep-learning architecture on vast amounts of region-specific content, empowering it to grasp cultural contexts and colloquialisms that other models often miss in translation.
Key Features of SUTRA
- Multilingual Mastery: SUTRA is equipped to handle more than 20 major Indian languages with remarkable fluency. This is significant in a country like India, where language changes every few hundred kilometers. SUTRA doesn’t just translate or interpret words—it understands them within the correct regional and cultural context.
- Contextual Accuracy: Understanding the local context is critical in many Indian languages. Words can have different meanings depending on the tone, regional context, or even the caste and social hierarchy. SUTRA excels in understanding these subtleties, making it more accurate for Indian languages than its counterparts.
- Localized Approach: Unlike GPT-4 and LLaMA 3.1, which are generalized language models trained on a vast array of global datasets, SUTRA focuses specifically on Indian languages and their associated datasets. It doesn’t treat Hindi the same way it treats Tamil or Telugu—it recognizes the unique grammatical structures, syntax, and cultural aspects of each language.
How Do GPT-4, Gemini, and LLaMA 3.1 Compare in Indian Languages?
While models like GPT-4, Gemini, and LLaMA 3.1 are highly proficient in languages like English, French, and Spanish, they fall short when faced with the complexities of Indian languages. These global models weren’t built with a deep understanding of local dialects and cultural nuances, making them less effective for businesses and services that need to cater to India’s diverse linguistic landscape.
Overview of GPT-4
GPT-4, developed by OpenAI, is one of the most powerful and advanced language models in the world. It boasts remarkable fluency in English and several other globally spoken languages. However, when it comes to Indian languages, GPT-4 struggles with the depth required to fully understand the nuances of regional dialects. While it can manage basic translations and provide relatively accurate responses, it doesn’t possess the level of contextual and cultural comprehension that SUTRA offers.
Gemini’s Role in AI Language Development
Gemini, a prominent AI language model, has made strides in real-time language processing and generation, excelling in scenarios that demand rapid responses and high-quality output. Developed with a focus on Western languages like English, it has become a widely adopted tool for content creation, translation, and conversational AI. However, when it comes to Indian languages, Gemini faces several challenges. Indian languages are complex, with diverse grammatical structures, multiple scripts, and a heavy reliance on context. These nuances make it difficult for non-specialized models like Gemini to provide accurate translations, context-aware responses, or nuanced conversational outputs.
While Gemini performs impressively with languages like English, it struggles to maintain the same level of precision in languages such as Hindi, Tamil, or Bengali. In particular, Gemini often fails to capture the distinct tonalities and cultural references embedded in Indian languages. Furthermore, Indian languages often involve code-switching—where speakers mix languages like Hindi and English in the same conversation. Gemini has not yet mastered the intricacies of this type of language blending. This limits its usefulness for regional applications that require a deep understanding of Indian vernaculars.
Overall, Gemini’s role in AI language development is noteworthy, but its shortcomings in understanding Indian languages highlight the need for more specialized models tailored to this rich linguistic landscape. Until further adaptations are made, models like Gemini will continue to face challenges in fully unlocking the potential of AI-driven language solutions for India.
Performance of LLaMA 3.1
LLaMA 3.1 has garnered attention in the AI community for its lightweight and highly efficient design, offering fast processing speeds and low computational requirements. This makes it an attractive option for applications where hardware resources are limited, allowing broader accessibility to AI-powered tasks. However, despite its strengths, LLaMA 3.1 falls short when addressing the complexities of Indian languages. Much like other globally recognized AI models, LLaMA 3.1 has been primarily optimized for major languages like English and does not cater well to the subtleties of Indian dialects or the variety of scripts used across the country.
Indian languages often involve grammatical intricacies that require a deep contextual understanding, something that LLaMA 3.1 has not been fully equipped to handle. For example, regional dialects, formal versus informal linguistic tones, and mixed-language conversations, such as the widely spoken Hinglish (a blend of Hindi and English), pose significant challenges for this model. LLaMA 3.1’s ability to interpret these languages falters, as it struggles with tone, cultural nuances, and context. The same lightweight design that makes LLaMA 3.1 efficient also limits its performance in understanding and processing the nuances of Indian linguistic diversity.
Despite its global strengths in quick language processing, LLaMA 3.1’s performance in Indian languages remains underdeveloped. It lacks the specificity and cultural awareness needed to fully engage with the country’s linguistic needs. Without significant refinement or localization, models like LLaMA 3.1 may continue to lag behind when it comes to accurately and meaningfully processing India’s diverse linguistic landscape.
Why SUTRA Outperforms Other Models in Indian Language Processing
SUTRA’s unparalleled success in Indian language performance can be attributed to its focus on cultural and linguistic intricacies that are often overlooked by other AI models. By tailoring its datasets and algorithms to better understand the unique challenges posed by Indian languages, SUTRA manages to surpass GPT-4, Gemini, and LLaMA 3.1 in both accuracy and usability.
Understanding the Indian Linguistic Landscape
India’s linguistic landscape is not just complex but also incredibly diverse. With 22 officially recognized languages and countless dialects, AI models must navigate varying sentence structures, grammatical rules, and scripts. This is where SUTRA shines—it understands that each Indian language comes with its own set of unique rules, and it adapts accordingly.
For example, Hindi has a subject-object-verb sentence structure, while Tamil uses a subject-object-verb word order but with more complex sentence constructions. SUTRA’s language processing algorithms are capable of interpreting these subtle differences, making it more accurate than global models that may apply a one-size-fits-all approach to language.
Key Innovations in SUTRA’s Design
SUTRA incorporates several cutting-edge innovations that set it apart from its competitors:
- Advanced NLP Algorithms: Natural Language Processing (NLP) lies at the heart of any language model, and SUTRA takes this to the next level with NLP algorithms tailored specifically for Indian languages. These algorithms are capable of not only understanding individual words but also how they relate to each other in a culturally appropriate manner.
- Multimodal Learning: SUTRA doesn’t just rely on text data; it also integrates multimedia inputs, such as audio and visual data, to enhance its language processing capabilities. This is especially helpful in regions where multiple languages and scripts coexist, and where spoken language can vary from written forms.
The Importance of Cultural Nuances in AI
While models like GPT-4 and LLaMA 3.1 excel in technical accuracy, they often fail to account for cultural nuances, which play a critical role in effective communication. For Indian languages, cultural understanding is just as important as linguistic accuracy.
How SUTRA Embraces Local Contexts
Consider this scenario: in certain parts of India, words or phrases that are perfectly acceptable in one region may be offensive or misunderstood in another. SUTRA understands these cultural distinctions. It adapts its responses based on the local context, ensuring that it communicates in a way that is both accurate and culturally sensitive. For example, a Bengali-speaking user might use phrases or idioms that are specific to their region. SUTRA can interpret these correctly, whereas a global AI model might provide an inaccurate or generic translation.
Real-World Applications of SUTRA in Indian Languages
The real power of SUTRA lies in its practical applications. From government services to education and customer support, SUTRA is making a significant impact in several sectors by bridging the language gap.
SUTRA’s Role in Government Services
India’s government has increasingly embraced digital solutions to improve public services. However, a significant challenge has been language barriers. Many government websites and services are available only in Hindi and English, leaving non-Hindi-speaking citizens at a disadvantage. With SUTRA, government services can offer more inclusive support, translating documents, offering customer service in regional languages, and even processing forms or applications with better accuracy.
SUTRA in Education and Content Creation
Education is another area where SUTRA shines. In a country where millions of students study in regional languages, having AI support for educational content is crucial. SUTRA can help create localized learning materials, translate textbooks, and offer tutoring assistance in native languages. Additionally, content creators can use SUTRA to generate articles, blogs, and media in various Indian languages, making digital content more accessible to local audiences.
Enhancing Customer Support with SUTRA
Customer support is where SUTRA has truly proven its value. Indian businesses, especially those operating in regional markets, require customer service representatives who can speak the local language. SUTRA can automate these interactions, ensuring that customers receive support in their native tongue without losing the human touch. This is where Onfra, a Visitor Management System (VMS) platform, could benefit significantly.
The Future of SUTRA: What Lies Ahead?
SUTRA’s journey has just begun, and it shows no signs of slowing down. As the demand for localized AI solutions grows, SUTRA is expected to continue evolving. Future versions will likely include even more dialects, enhanced contextual understanding, and additional multimedia capabilities. By expanding its database and refining its algorithms, SUTRA aims to become the gold standard for Indian language processing in both the public and private sectors.
Conclusion
SUTRA has taken the world by storm by mastering what other global models have struggled with—Indian language processing. By focusing on cultural nuances, contextual accuracy, and localized datasets, SUTRA has surpassed even the most renowned AI models like GPT-4, Gemini, and LLaMA 3.1 in this domain. Its real-world applications in sectors like education, government services, and customer support, along with its potential to enhance platforms like Onfra, make SUTRA a game-changer for India’s multilingual environment.
FAQs
- What makes SUTRA stand out from other AI models?
SUTRA excels in Indian language performance by focusing on regional dialects, cultural nuances, and localized datasets, something global models like GPT-4 and Gemini lack. - Can SUTRA handle all Indian languages?
SUTRA currently supports over 20 Indian languages, and it is continually evolving to include more dialects and regional variations. - How does SUTRA benefit Onfra’s services?
SUTRA enhances Onfra’s visitor management platform by providing multilingual support, making it more accessible to a broader audience across India. - Are there any limitations to SUTRA?
While SUTRA is highly proficient in Indian languages, it may not be as advanced in handling less commonly spoken dialects or non-Indian languages. - Will SUTRA continue to improve?
Yes, SUTRA is expected to keep evolving, expanding its language capabilities and refining its contextual accuracy to meet future needs.
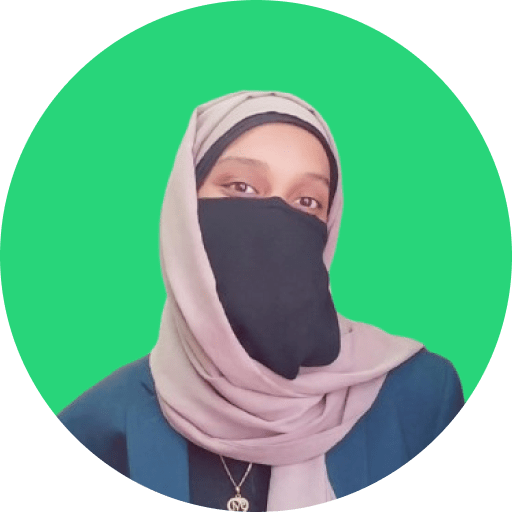
A subject matter expert in facilities, workplace, culture, tech, and SaaS, I create impactful content strategies that enhance startup retention and foster strong connections. With a blend of technical expertise and creativity, I drive engagement and loyalty. Always eager for challenges and make a lasting impact.